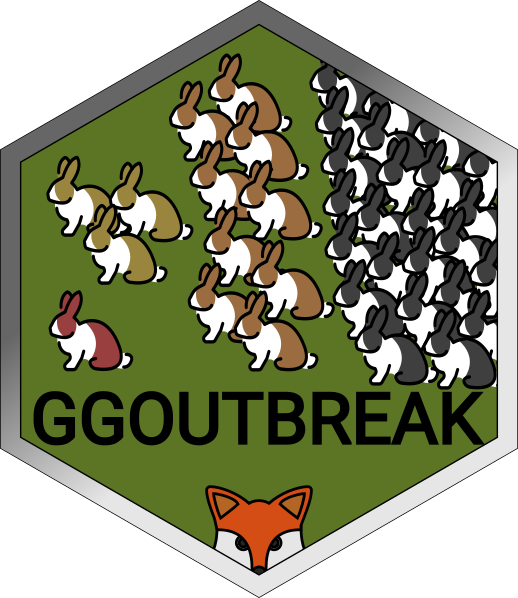
Augment a line list of infection with a set of events
Source:R/simulation-utils.R
sim_apply_delay.linelist.Rd
Events include symptom onset, admission, death, test sampling, test processing
Usage
sim_apply_delay.linelist(
df = i_sim_linelist,
...,
fn_p_symptomatic = ~0.5,
fn_symptom_delay = ~rgamma2(.x, mean = 5),
fn_p_admitted = ~0.1,
fn_admission_delay = cfg_weekly_gamma_rng(c(8, 8, 8, 8, 8, 9.5, 9)),
fn_p_died = ~0.05,
fn_death_delay = ~rgamma2(.x, mean = 14),
fn_p_tested = ~0.8,
fn_sample_delay = cfg_weekly_gamma_rng(c(1, 1, 1, 1, 1, 1.5, 1.4)),
fn_result_delay = cfg_weekly_gamma_rng(c(1, 1, 1, 1, 1, 1.6, 1.5)),
seed = Sys.time()
)
Arguments
- df
a line list dataframe arising from e.g.
sim_branching_process()
- a dataframe with columns:id (unique_id) - Patient level unique id
time (ggoutbreak::time_period) - Time of infection. A `time_period`
Any grouping allowed.
- fn_p_symptomatic, fn_p_admitted, fn_p_died, fn_p_tested
Function that returns a probability between 0 and 1 for each row of the input dataframe. A
purrr
style lambda is OK (e.g.~ 1
for always true) the first parameter of this will be time of infection. The function must be vectorised on its inputs (and consume additional inputs with...
)- fn_symptom_delay, fn_admission_delay, fn_death_delay,
a function that calculates the time to event onset from infection. This will be called with a vector of infection times as the first parameter (
time
) but all other columns ofdf
are also available as well as thesymptomatic
,died
,andadmitted
flags. The function must be vectorised on its inputs (and consume additional inputs with...
). Apurrr
style lambda is OK e.g.~ stats::rgamma(.x, shape = 3)
, and the first parameter will be infection time. if you have an discrete probability profile for this then you can usecfg_ip_sampler_rng(ip_symptoms)
.- fn_sample_delay
This function returns the time from either symptom onset (symptomatic) or from infection (asymptomatic) until a sample is taken. (N.B. this might be better to do a screening test probability plus screening test frequency rather than overloading this.)
- fn_result_delay
Identical to other functions except the first parameter will be
sample_time
rather than time of infection. This is the time from sampling to the result being available.- seed
RNG seed for reproducibility
Examples
tmp = sim_branching_process(
changes = tibble::tibble(t = c(0,20,40,60,80,110), R = c(1.8,1.5,0.9,1.5,0.8,1.2)),
max_time = 120,
seed = 100
)
#> .
#> .
#> .
#> .
#> .
#> .
#> .
#> .
#> .
#> .
#> .
#> .
#> .
#> .
#> .
#> .
#> .
#> .
#> .
#> .
#> .
#> .
#> .
#> .
#> .
#> .
#> .
#> .
#> .
#> .
#> .
#> .
#> complete
tmp2 = tmp %>% sim_apply_delay()
tmp2 %>% dplyr::glimpse()
#> Rows: 42,202
#> Columns: 20
#> $ time <time_prd> 0, 0, 0, 0, 0, 0, 0, 0, 0, 0, 0, 0, 0, 0, 0, …
#> $ id <int> 1, 2, 3, 4, 5, 6, 7, 8, 9, 10, 11, 12, 13, 14, 15,…
#> $ generation_interval <dbl> NA, NA, NA, NA, NA, NA, NA, NA, NA, NA, NA, NA, NA…
#> $ infector <int> NA, NA, NA, NA, NA, NA, NA, NA, NA, NA, NA, NA, NA…
#> $ generation <dbl> 0, 0, 0, 0, 0, 0, 0, 0, 0, 0, 0, 0, 0, 0, 0, 0, 0,…
#> $ symptom <lgl> FALSE, FALSE, FALSE, FALSE, TRUE, FALSE, FALSE, FA…
#> $ symptom_delay <dbl> NA, NA, NA, NA, 5.065803, NA, NA, NA, NA, 6.735023…
#> $ symptom_time <time_prd> NA, NA, NA, NA, 5.065803, NA, NA, NA, NA, 6.7…
#> $ admitted <lgl> FALSE, FALSE, FALSE, FALSE, FALSE, FALSE, FALSE, F…
#> $ admitted_delay <dbl> NA, NA, NA, NA, NA, NA, NA, NA, NA, NA, NA, NA, NA…
#> $ admitted_time <time_prd> NA, NA, NA, NA, NA, NA, NA, NA, NA, NA, NA, N…
#> $ death <lgl> FALSE, FALSE, FALSE, FALSE, FALSE, FALSE, FALSE, F…
#> $ death_delay <dbl> NA, NA, NA, NA, NA, NA, NA, NA, NA, NA, NA, NA, NA…
#> $ death_time <time_prd> NA, NA, NA, NA, NA, NA, NA, NA, NA, NA, NA, N…
#> $ tested <lgl> TRUE, FALSE, FALSE, TRUE, TRUE, TRUE, FALSE, TRUE,…
#> $ sample_delay <dbl> 0.27126683, NA, NA, 0.98555013, 1.16229296, 1.3646…
#> $ sample_time <time_prd> 0.27126683, NA, NA, 0.98555013, 1.16229296, 1…
#> $ result <lgl> TRUE, TRUE, TRUE, TRUE, TRUE, TRUE, TRUE, TRUE, TR…
#> $ result_delay <dbl> 0.32735508, 0.52412293, 0.60239350, 1.09007272, 1.…
#> $ result_time <time_prd> 0.59862191, NA, NA, 2.07562285, 2.43786808, 2…